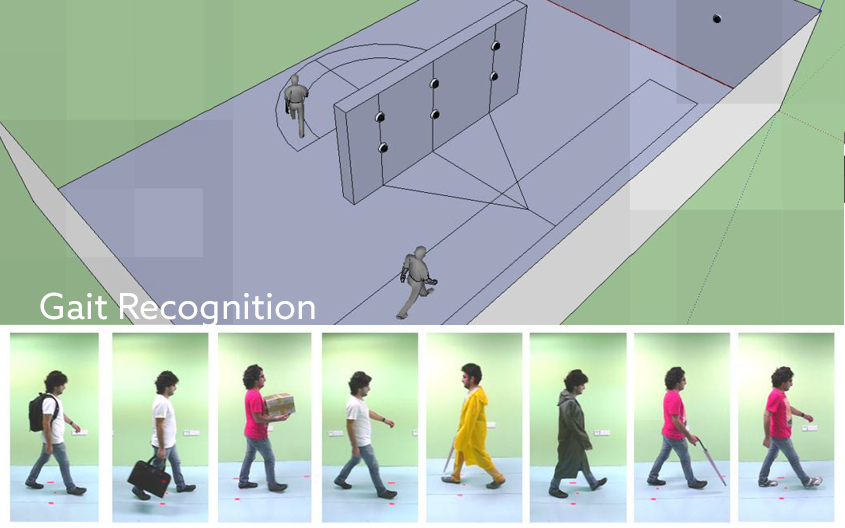
Gait Recognition for Automated Visual Surveillance and Security
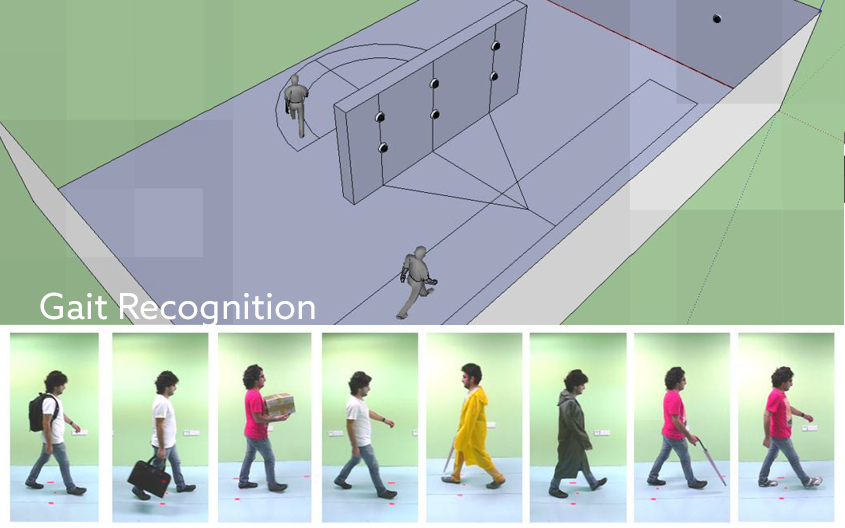
The security of high-privileged premises like self-service bank and company safety vault is very crucial. In reality, many robberies and violent events have occurred at these premises and resulted in great financial losses and physical injuries. If these events can be instantly detected and controlled, the resulting damages could be reduced. However, as the majority of the present video surveillance systems cannot automatically detect these events, they are not able to stop the violent incidents or reduce the damages incurred. In this respect, gait recognition appears as an attractive solution to this problem. Gait recognition is used to signify the identity of a person based on the way the person walks. This is an interesting property to recognize a person, especially in surveillance or forensic applications where other bio-metrics may be inoperable. For example in a bank robbery, it is not possible to obtain face or fingerprint impressions when masks or hand gloves are worn. Therefore, gait appears as an attractive solution because gait is discernible even from a great distance.
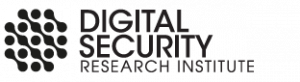
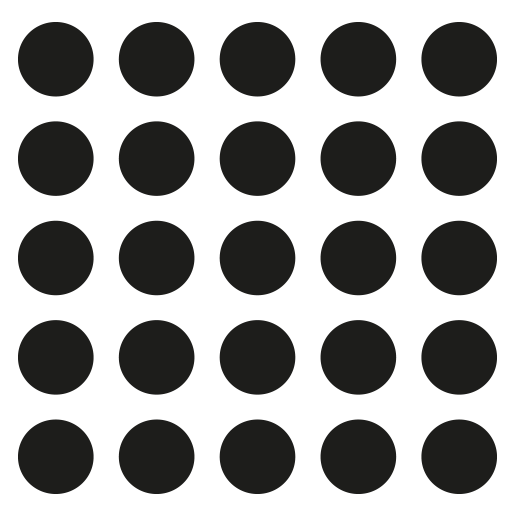
he MMU Digital Futures Research Hub is a multi-disciplinary platform for research on the digitalization of our society. The goal is to foster innovative, cutting-edge multi-disciplinary research, and to provide outstanding training for talented young scholars and students through 8 research institutes.
The hub is a community and industry centric entity, with 20 professor chairs that brings together universities, governmental and industrial research organizations, as well as state and federal governments.