Medical Images Classification through Deep Neural Networks
In year 2019, 268,600 new invasive type malignancy breast cancer cases are estimated will be developed in women, while 62,930 new non-intrusive breast cancer disease will be diagnosed in women (Breast cancer statistics, 2019). In Malaysia, women have one of every 19 chances to be diagnosed of this dreaded disease during her lifetime (FreeMalaysiaToday, 2019; Chen & Molloi, 2003). Over the globe, there is a woman being diagnosed with breast cancer disease somewhere in the world in every 15 seconds and more than six women die of breast cancer every five minutes worldwide (New Strait Times, 2019). Of all major races in Malaysia, Chinese women have highest risk (6.25%) to be diagnosed as being breast cancer patient during her lifetime. In comparison, lifetime danger of Indian and Malays women are 5.88% and 3.57% respectively (FreeMalaysiaToday, 2019).
As suggested by studies, malignancy’s phase during diagnosis highly affected the breast cancer survival rate (Youlden et al., 2012). In order to decrease the dismalness and death rate from this disease, an early detection is needed for medical experts to provide appropriate treatment to breast cancer patient. An informative diagnosis for various cancer classification is crucial to assist medical experts for selection of legitimate treatment (Chen et al., 1995). Women under 50 years old have experienced larger decreases of their risk of being diagnosed as breast cancer patients. The technology advances on treatment, early detection through screening test and improved awareness on breast cancer among women are suggested as the reasons for this phenomenon (Breast cancer statistics, 2019).
Traditionally, physicians need to manually delineate the suspected breast cancer area. Numerous studies have mentioned that manual segmentation takes time, and depends on the machine and the operator. Recent years, many research studies utilised deep learning and artificial intelligence to solve medical imaging issues. Artificial Neural Network (ANN) provides instruction for trained model to perform the assigned task instead of preconfigured the system to execute the assigned tasks.
In year 2017, Centre for e-Health were funded by FRGS for breast cancer classification through neural networks project. The algorithm called Convolutional Neural Network Improvement for Breast Cancer Classification (CNNI-BCC) is presented to assist medical experts in breast cancer diagnosis in timely manner. The CNNI-BCC uses a convolutional neural network that improves the breast cancer lesion classification in order to help experts for breast cancer diagnosis. CNNI-BCC can classify incoming breast cancer medical images into malignant, benign, and healthy patients. The application of present algorithm can assist in classification of mammographic medical images into benign patient, malignantpatient and healthy patient without prior information of the presence of a cancerous lesion. The presented method aims to help medical experts for the classification of breast cancer lesion through the implementation of convolutional neural network for the classification of breast cancer. CNNI-BCC can categorize incoming medical images as malignant, benign or normal patient with sensitivity, accuracy, area under the receiver operating characteristic curve (AUC) and specificity of 89.47%, 90.50%, 0.901 ± 0.0314 and 90.71% respectively.
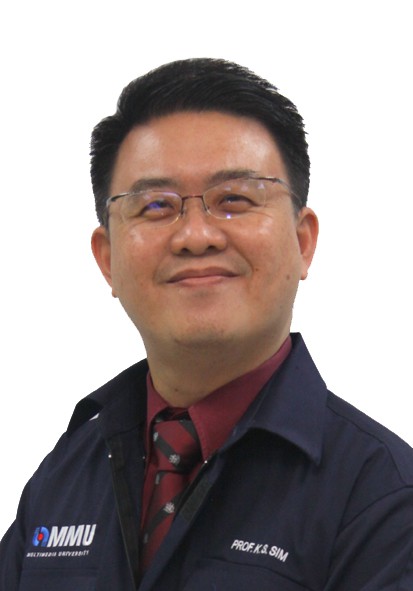
Professor Dr. Ir. Sim Kok Swee and his research team in medical imaging and biomaterials have developed robust methodologies to detect breast cancer, early infarct, endoscopy and tuberculosis. In addition, his team also developed new techniques for signal to noise ratio (SNR) measurement. When interacting with scanning electron microscopy (SEM), these techniques enable auto real-time noise quantization and self-improvement for SEM image quality. Another important contribution by his team is in prediction of signal as well as noise from the images. This enhances the analysis skills to study the image mechanism at nano-scale level.